The Complete Guide To Launching Your Data Analytics Operations
In today’s business world, harnessing the power of data and analytics is a key competitive advantage for companies of all sizes and industries. Most organizations understand the value of capturing customer data and leveraging these insights towards better decision making and streamlined workflows and operations. But Data Analysts know that getting to this point — when you can truly say you work for a data-driven business — takes time, effort, and practice.
So where do you begin?
From setting up your data infrastructure to growing your team and building out your data strategy, there are a number of important steps towards achieving your data goals. This complete guide breaks down the core components of data analytics operations, to help you tackle them one at a time and move towards data maturity.
What does Data and Analytics mean?
First and foremost: when we talk about your company’s data analytics operations, what do we really mean? Your data and analytics operations encompass everything you do to collect, store, analyze, and activate your business data. Essentially, it’s how you take your raw data, turn it into actionable insights, and translate that information into strategic decisions, initiatives, or optimizations. As more and more organizations embrace data analytics, it’s becoming a central part of how many companies function.
97% of business leaders said they’re investing in data initiatives in 2022, and 60% expect their companies to become data-driven in the next 2-3 years.
– NewVantage Partners’ 2022 Data and AI Executive Survey
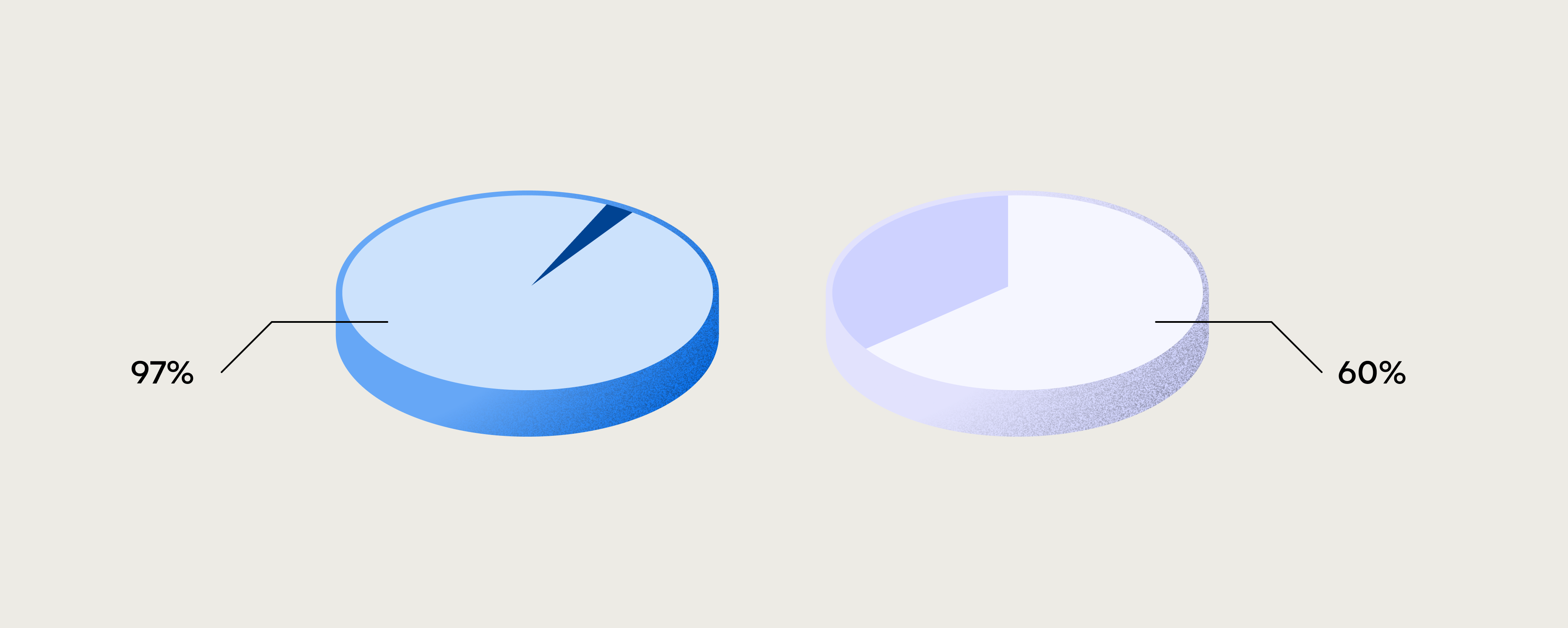
But becoming data-driven is about more than gathering insights. It’s about what you do with those insights, be it through data visualization, building reverse-ETL pipelines, or automating core tasks. Modern companies expect to take their data operations beyond the analytics that got them to where they are today, and into the data activation that will set them ahead of the competition of tomorrow.
So whether you’re launching data analytics operations at a startup or working to modernize the way data is used at a larger, more established organization, it’s an exciting time to be in the data field. But, you’ve also got your work cut out for you — so keep reading and take it one step at a time.
Building your data infrastructure
Launching your company’s data analytics operations requires a solid infrastructure, starting with a data warehouse to centralize your company data. You also need the right tools in place to extract, load, transform, model, visualize, and activate your data. In other words, you need a Modern Data Stack.
What is a Modern Data Stack?
As data operations have advanced and become more commonplace, the tooling around data has also evolved. In recent years, there’s been an emergence of new software to perform various tasks related to data analytics, from ELT to reverse-ETL, modelling, data visualization tools, and more. Together, these niche tools have come to be known as a ‘Modern Data Stack’, and each stack tends to be a little different based on the needs of the data team and the context of the business.
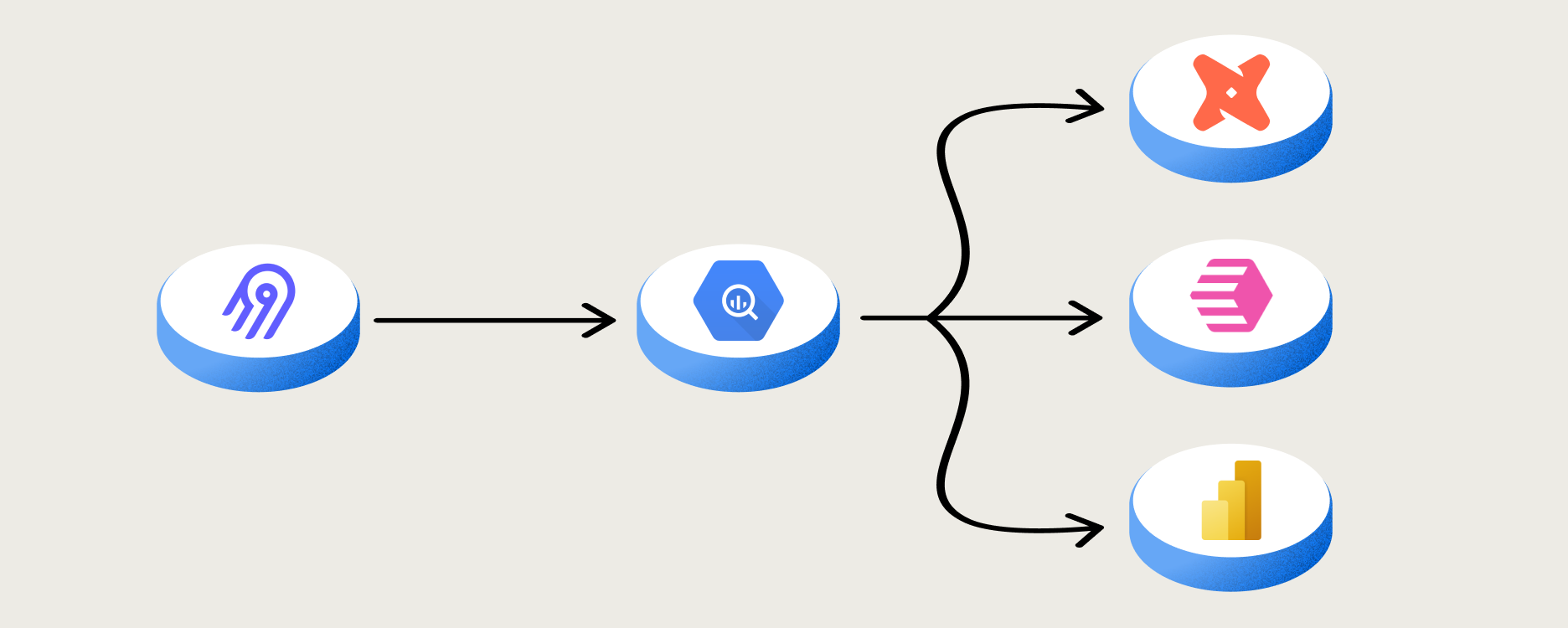
The problem with the Modern Data Stack
While the emergence of the Modern Data Stack has been an important step forward in enabling data teams, what was once considered to be a ‘modern’ stack has quickly become the new legacy stack. Complex systems to patch tools together have led to inefficiencies as teams struggle to maintain their pipelines and troubleshoot problems as they arise. Simply put, the Modern Data Stack hasn’t scaled with the rapid advancement of analytics operations.
The future of the Modern Data Stack
Modern challenges call for modern solutions, and innovation in data tooling hasn’t slowed down. Having experienced the difficulties of maintaining a so-called modern data stack first-hand, the team at Weld started to rethink what kind of tooling Data Analysts really need today. And this is how the Weld platform has grown to what it is today: a single place for all of your core data operations.
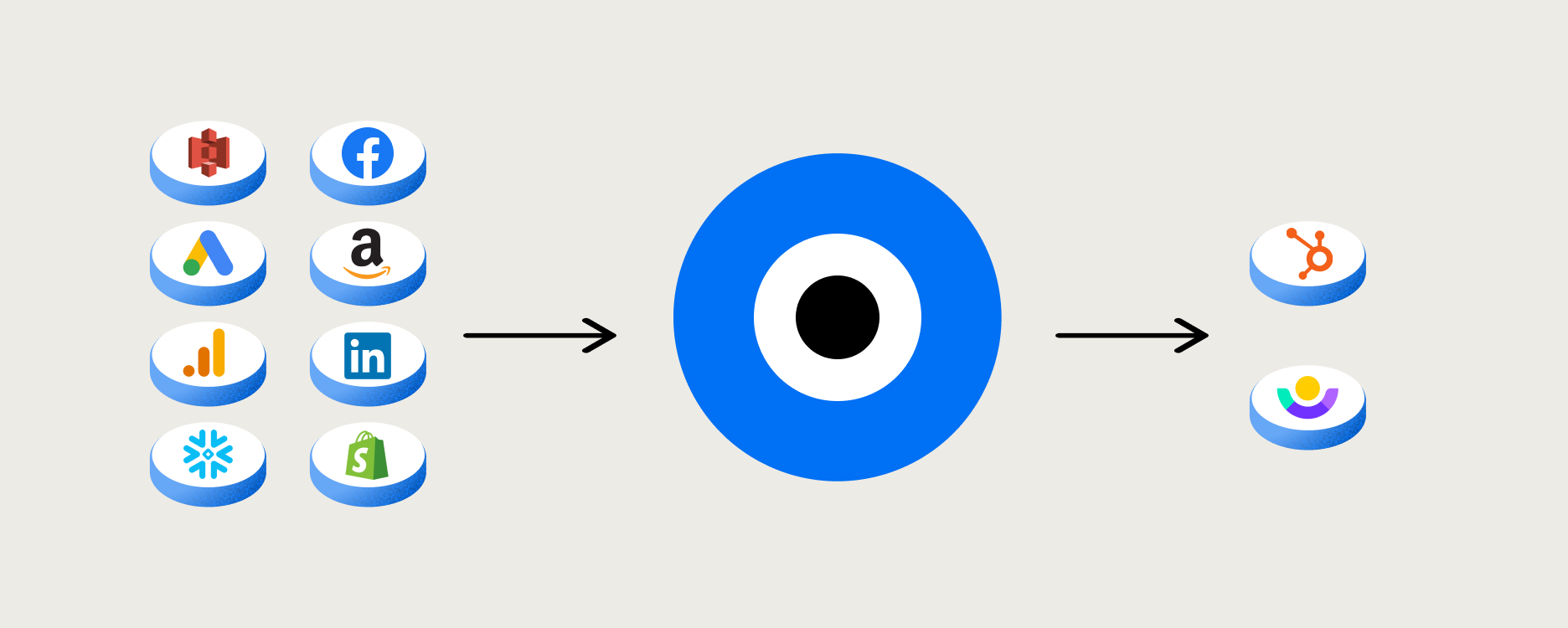
For organizations ready to get up and running with their data operations, Weld is a solid foundation to build on without the need for multiple tools, a large data team, or custom scripts. You can perform your ELT and reverse-ETL with dozens of pre-built connectors, and centralize all of your models in the same space. Built on top of your data warehouse, it’s a simple, fast, and scalable solution for businesses of all sizes and industries.
Curious to see how Weld can power your data operations? Sign up free today, or book a call with one of our specialists.
Creating your data strategy: 4 key steps
Like any central business domain, your data analytics efforts are going to need a strategy to follow. A clearly outlined strategy helps every member of the team — including stakeholders and non-technical experts — really grasp what it is you’re working towards.
Just 53% of companies surveyed report that they have formulated a corporate data strategy to realize business value.
– NewVantage Partners’ 2022 Data and AI Executive Survey
Alignment and clarity are essential to a data specialist’s success. A common understanding makes it much easier to make decisions and work collaboratively with a shared sense of purpose and direction. And, it creates a point of reference for the impact of all your hard work.
1. Set your data goals and objectives
Clear strategies start with clear objectives. Depending on your organizational structure, you might team up with your company leadership or fellow data team members to craft your goals, or it could be an individual task. And depending on the stage, size, and ambitions of the business, the types of goals you’ll want to achieve through your data operations will look different, too.
Gather information by speaking with stakeholders, referring to strategic documentation, and looking at high-level company goals and OKRs. With these insights, you can set some specific goals and objectives you want to achieve through your data efforts. Be sure your goals are clear, measurable, and realistic.
Examples of data goals
- Establish the data warehouse as a single source of truth for company data
- Map the customer lifecycle to inform the customer success strategy
- Build automated reports for all commercial teams
2. Define your core metrics
It might not be one of the goals you outline, but it’s still essential that you clearly define your core business metrics, and share these definitions throughout the organization. Too often — especially as companies scale — the metrics that help you measure performance start to take on different meanings for different teams or departments. You want people to be on the same page when they discuss CAC, MRR, or LTV. In other words, you want to create KPI alignment across teams.
How to define your core business metrics
- Ask teams for their input: Gather information from every team on which metrics they track and how they measure them, to give you a clear overview of how things are currently being done.
- Select your most important metrics: Looking at the metrics that are already being tracked and spotting any holes, create a list of the core metrics you want to define at a company level.
- Set up your tracking and create definitions: Establish how you’ll track these metrics and make sure there’s clear lineage for their technical definitions. Then, create commercial definitions so every team has a common understanding.
When it comes to business metrics, having a central source of truth is so important for keeping everyone aligned. Weld’s Metrics Store brings your metrics definitions into the same platform as the rest of your data operations. Clear lineage, change logs, and commercial definitions mean everyone has a shared understanding of how each metric is calculated and measured.
3. Establish data governance
Once you have clearly defined goals and metrics definitions, it’s important to put systems and processes in place to keep your data secure, accurate, and accounted for. Creating data governance means establishing norms for how company data is accessed, used, and monitored across the organization. This includes establishing data ownership of specific KPIs or other metrics.
Data governance structures you can implement
- Clearly outlined policies around the proper use and protection of company data.
- An organizational chart that indicates which teams are accountable for which data.
- Proper tooling so staff can access, interpret, and use data in their function.
Data governance isn’t just about accountability, it’s also about data quality and accessibility. Respondents to McKinsey’s 2019 Global Data Transformation Survey reported an average of 30% of total enterprise time spent on non-value-added tasks because of poor data quality and availability. It’s the data team’s responsibility to make sure data consumers in the organization have access to clear, reliable data so they can uphold their data responsibilities.
One of the best ways to improve data accessibility is with simple, intuitive tooling. Bringing your core data operations into a single platform can make a world of difference not just for your data team’s efficiency, but also for how other members of the company interact with (and trust) your customer data. Weld centralizes your data operations and offers an easy-to-navigate experience for those who are less experienced with data. Give it a try.
4. Develop data literacy across departments
Getting clear, reliable data into the right hands is one thing — but you also need to equip people to understand and use it. Forrester and Tableau found that while 82% of leaders expect all employees to have basic data literacy, only 47% of employees say they’ve been offered data training by their organization. Supporting employees across departments to interact with data and use it in their day-to-day is a key piece of a strong data strategy.
There are a number of ways you can help build data literacy across your organization, and the steps outlined above (setting your data goals, defining your metrics, and establishing data governance) are all great ways to start. To take it a step further, be sure to communicate all of this clearly and update people frequently. The more people hear about the role data plays at the organization, the more familiar and comfortable they’ll be with it.
Ways to improve data literacy for non-technical employees
- Host training sessions to educate employees on the basics of data analysis, and make it a workshop where they can practice interpreting real company data in groups.
- Get departments to examine the performance of their initiatives at a monthly review meeting, and discuss how they can apply their learnings going forward.
- Create documentation on the best practices for accessing and using data at your company, and make it easily available through your intranet.
How to build a data analytics team
Alright, we’ve covered a lot of the technical stuff…but what about building an all-star data team to keep it all running? Launching your data operations usually at least means hiring a data analyst (if your company doesn’t yet have one), but could even mean hiring a big team of data engineers, data analysts, and data scientists. It will really depend on the stage of your company and the data ambitions you have.
Start by getting clear on what kind of expertise or experience you need to fulfill your goals. If you’re a solo data specialist looking to build your team, do you need a co-pilot with a similar skill set to you, or someone with a different background who can help you branch out in your work? This will help you narrow your search and create job postings that really reflect the unique type of candidate you’re looking for.
Keep in mind that with the innovation that’s been taking place in the data tooling space, it’s no longer a requirement to have a 10+ person team of experts to start deriving value from your company data. With an end-to-end data tool like Weld, you can easily get up and running in a matter of hours, with or without a data team. For teams of one or two people, it can set you months or even years ahead in getting your operations off the ground.
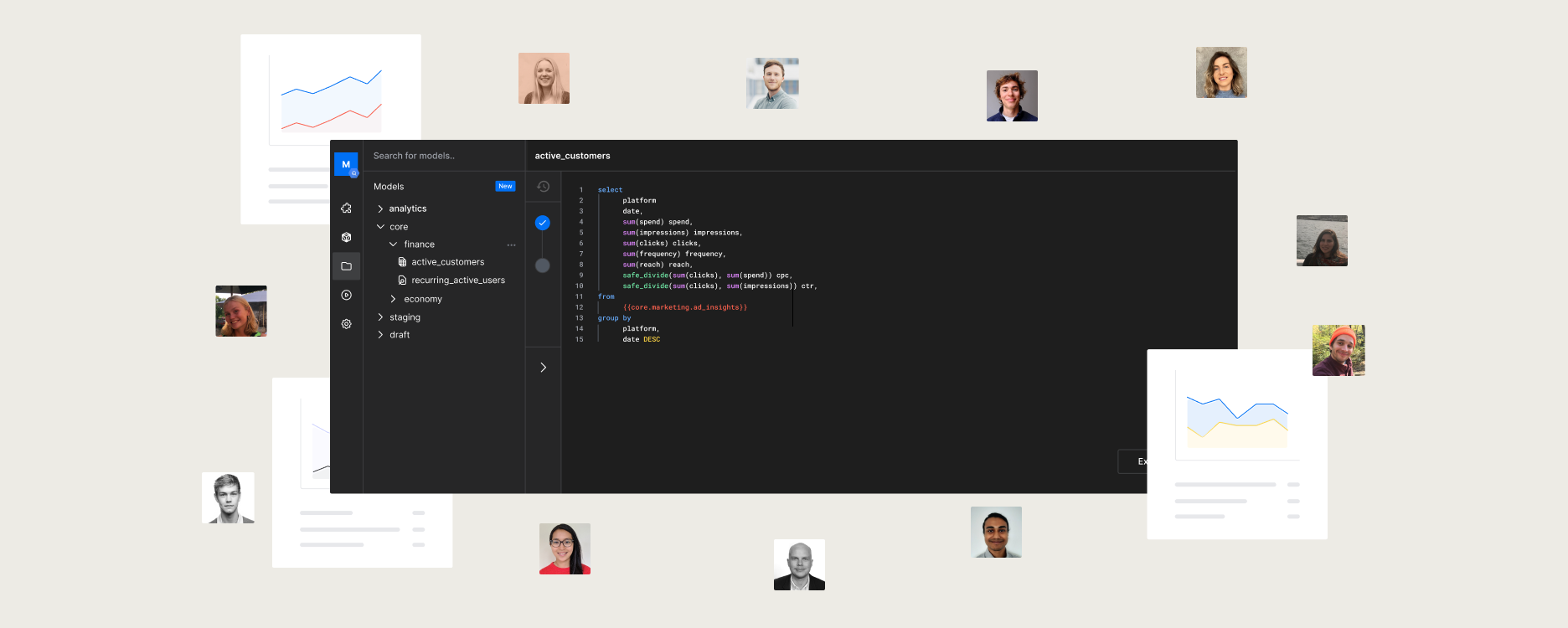
Not only that, Weld offers different pricing tiers to fit your needs, and each comes with dedicated onboarding hours from our in-house team of expert Data Analysts. As one customer recently shared on Product Hunt:
“Before deciding to build our BI capabilities with Weld I evaluated a number of other options and in the end determined Weld to be the best both because the tool enabled us to have the flexibility and robustness we needed to define our metrics and add new data sources in the future, and I knew we could rely on a strong data engineering team from Weld to help set everything up.”
Every business can leverage the power of data
The Netflix algorithm wasn’t built in a day. Developing your company’s data analytics operations will take some time, but unlike the data landscape of yesteryear, it’s truly possible for organizations of all sizes and industries to tap into the power of their data. Use this guide as a framework for getting started, and lay the foundation that will help you achieve all of your ambitious data dreams.